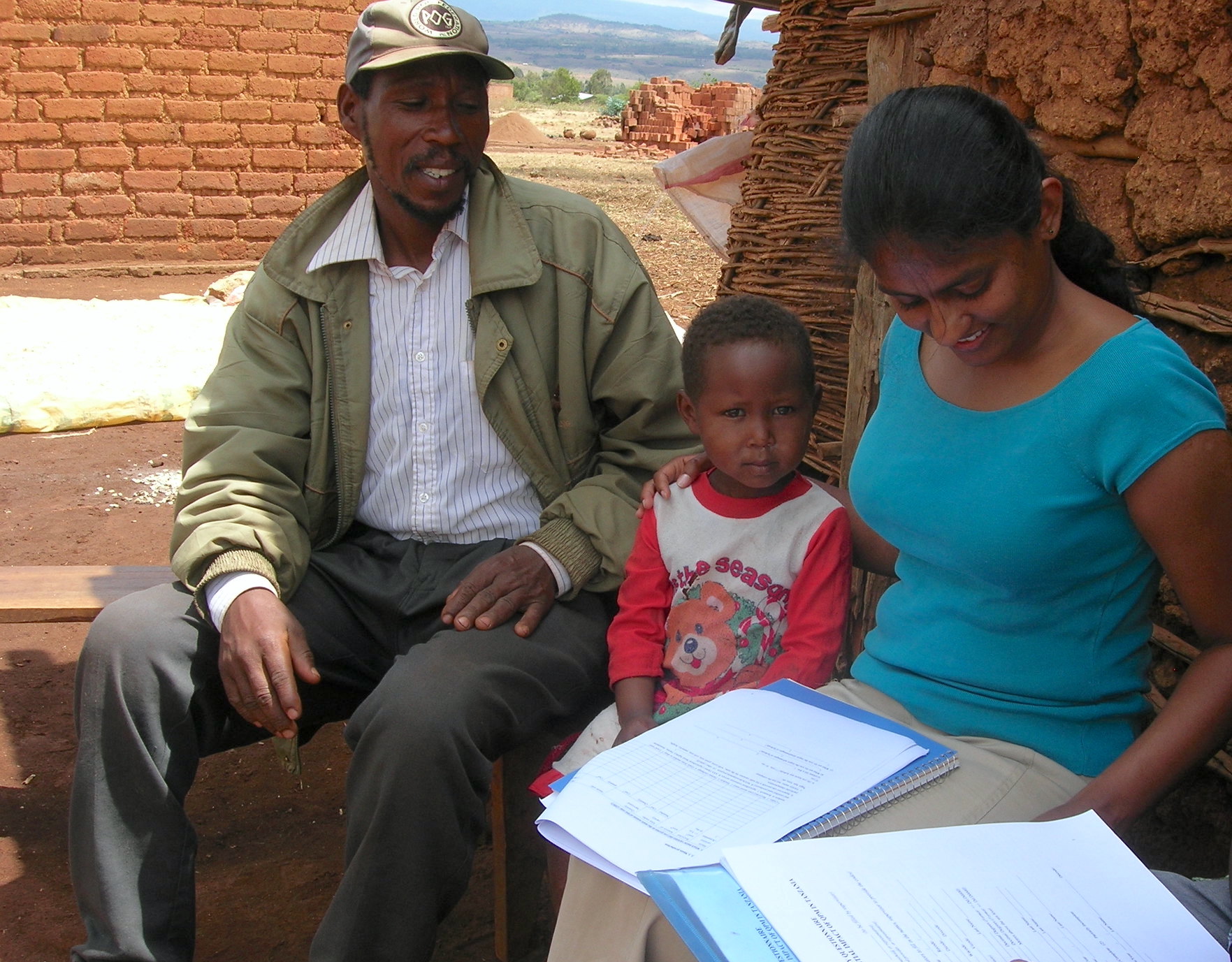
Recently, I published the technical description of Ontology-Agnostic Metadata Schema (OIMS) in the journal Frontiers in Sustainable Food Systems, as part of a special issue on “Agile Data-Oriented Research Tools to Support Smallholder Farm System Transformation.”
CGIAR and the International Maize and Wheat Improvement Center (CIMMYT) are dedicated to providing research data information products (RDIP) in open access, following the FAIR data standards. FAIR stands for findable, accessible, interoperable and reusable. Organizations dedicated to open data have made massive progress in making data findable and accessible. A clear example is a free, open-access repository of research studies developed by CIMMYT scientists. Article 4.1.c.i. of the CGIAR data policy states that “Relevant data assets (e.g. datasets) and metadata shall be interoperable and fit for reuse.”
This is easier said than done. There are well-established standards for descriptive metadata such as the Dublin Core and the derived standard used widely across the CGIAR, aptly called CGcore, used in CIMMYT’s Dataverse research data repository. However, these standards are lacking in many domains for describing the actual content of data sets.
At best, idiosyncratic data dictionaries are developed for specific datasets, projects and sometimes even programs. Idiosyncratic data dictionaries help make data interoperable but, in many cases, require a lot of preprocessing before scientists can actually reuse the data. Having a standard for data dictionaries would be a huge leap forward, but is not likely to happen anytime soon.
The next best thing is to standardize the way that you describe data dictionaries. This was recognized by the community of practice on socioeconomic data of the CGIAR Platform for Big data in Agriculture. Over the past few years, efforts led by CIMMYT set to remedy that lack of a standard, resulting in the flexible, extensible, machine-readable, human-intelligible and ontology-agnostic metadata schema (OIMS).
The paper in the journal Frontiers in Sustainable Food Systems describes a lightweight, flexible, and extensible metadata schema. It is designed to succinctly describe data collected for international agricultural research for development, facilitating interoperability. The schema is also meant to make it easier to store, retrieve and link different datasets stored in a data lake.
Agricultural research data comes to the surface
The paper discusses a need for this type of schema. Typically, agricultural research data comes in different formats and from different sources. For example, we can have structured surveys, semi-structured surveys, mobile phone records and satellite data. In the case of socioeconomic data, it can be particularly “messy.” To facilitate interoperability, we need to find methods to describe these datasets, which are machine readable — or actionable.
There have been other attempts to provide a standardized way to make data interoperable. Past approaches have been comprehensive but cumbersome. That could be the reason why they are typically only used by larger-scale projects. OIMS provides a framework that can be used by all data managers and scientists to enhance the interoperability for research data to ensure the data can be reused with much more ease.
The paper provides a detailed description of OIMS, including: the metadata schema, which describes the data dictionary; and the self-describing metadata, which describes the fields in the metadata. The paper then demonstrates the utility of this schema using a small segment of a household survey.
This paper presents an internally consistent approach to providing metadata for data files when standards are missing. It is flexible and extensible, so it will not be obsolete before it is implemented at scale. The approach is based on the concept of data lakes where data is stored as is. To ensure that data lakes do not become swamps, metadata is indispensable. The OIMS metadata schema approach can help to standardize the description of metadata and thus can be considered the fishing gear to extract data from the data lake.
As part of the on-going work started by the community of practice on socioeconomic data of the CGIAR Platform for Big Data in Agriculture, implementation of the OIMS metadata schema approach on datasets that can create indicators highlighted in the 100Q approach with linkages to the nascent socioeconomic ontology SEOnt is envisaged. This will provide datasets with enhanced interoperability.
With more and more datasets using the OIMS approach in the near future, it will become possible to turn what is currently a socioeconomic data swamp into a data lake. This will provide timely actionable information to support agri-food systems transformation — helping smallholders make a living while staying within planetary boundaries.
Implementing OIMS in practice requires data managers and scientists that collect the data to actively engage in providing the relevant metadata. As mentioned before, some of the metadata can be gleaned from the software solutions the scientists use already. As these are structured metadata, they can be extracted by machines. Often it does require curation by the scientist involved, especially when the software solution does not provide key information that the scientist has at hand but is not documented in a machine-readable way.
Read the full paper:
A Flexible, Extensible, Machine-Readable, Human-Intelligible, and Ontology-Agnostic Metadata Schema (OIMS)